AI that mimics human problem solving is a big advance – but comes with new risks and problems
Central to this is the model’s ability to employ “chain-of-thought reasoning” – a method similar to a human using a scratchpad, or notepad, to write down intermediate steps when solving a problem

Estimated reading time: 7 minutes
OpenAI recently unveiled its latest artificial intelligence (AI) models, o1-preview and o1-mini (also referred to as “Strawberry”), claiming a significant leap in the reasoning capabilities of large language models (the technology behind Strawberry and OpenAI’s ChatGPT). While the release of Strawberry generated excitement, it also raised critical questions about its novelty, efficacy and potential risks.
Chain-of-thought reasoning mirrors human problem solving by breaking down complex tasks into simpler, manageable sub-tasks. The use of scratchpad-like reasoning in large language models is not a new idea.
The ability to perform chain-of-thought reasoning by AI systems not specifically trained to do so was first observed in 2022 by several research groups. These included Jason Wei and colleagues from Google Research and Takeshi Kojima and colleagues from the University of Tokyo and Google.
Before these works, other researchers such as Oana Camburu from the University of Oxford and her colleagues investigated the idea of teaching models to generate text-based explanations for their outputs. This is where the model describes the reasoning steps that it went through in order to produce a particular prediction.
Even earlier than this, researchers including Jacob Andreas from the Massachusetts Institute of Technology explored the idea of language as a tool for deconstructing complex problems. This enabled models to break down complex tasks into sequential, interpretable steps. This approach aligns with the principles of chain-of-thought reasoning.
Strawberry’s potential contribution to the field of AI could lie in scaling up these concepts.
A closer look
Although the exact method used by OpenAI for Strawberry is shrouded in mystery, many experts think that it uses a procedure known as “self-verification”.
This procedure improves the AI system’s own ability to perform chain-of-thought reasoning. Self-verification is inspired by how humans reflect and play out scenarios in their minds to make their reasoning and beliefs consistent.
Most recent AI systems based on large language models, such as Strawberry, are built in two stages. They first go through a process called “pre-training”, where the system acquires its basic knowledge by running through a large general dataset of information.
They can then undergo fine-tuning, where they are taught to perform specific tasks better, typically by being provided with additional, more specialised data.
This additional data is often curated and “annotated” by humans. This is where a person provides the AI system with additional context to aid its understanding of the training data. However, Strawberry’s self-verification approach is thought by some to be less data-hungry. Yet, there are indications that some of the o1 AI models were trained on extensive examples of chain-of-thought reasoning that have been annotated by experts.
This raises questions about the extent to which self-improvement, rather than expert-guided training, contributes to its capabilities. In addition, while the model may excel in certain areas, its reasoning proficiency does not surpass basic human competence in others. For example, versions of Strawberry still struggle with some mathematical reasoning problems that a capable 12-year-old can solve.
Risks and opacity
One primary concern with Strawberry is the lack of transparency surrounding the self-verification process and how it works. The reflection that the model performs upon its reasoning is not available to be examined, depriving users of insights into the system’s functioning.
The “knowledge” relied upon by the AI system to answer a given query is not available for inspection either. This means there is no way to edit or specify the set of facts, assumptions, and deduction techniques to be used.
Consequently, the system may produce answers that appear to be correct, and reasoning that appears sound, when in fact they are fundamentally flawed, potentially leading to misinformation.
Finally, OpenAI has built in protections to prevent undesirable uses of o1. But a recent report by OpenAI, that evaluates the system’s performance, did uncover some risks. Some researchers we have spoken to have shared their concerns, particularly regarding the potential for misuse by cyber-criminals.
The model’s ability to intentionally mislead or produce deceptive outputs – outlined in the report – adds another layer of risk, emphasising the need for stringent safeguards.
Pasquale Minervini, Lecturer in Natural Language Processing, University of Edinburgh; Edoardo Ponti, Lecturer in Natural Language Processing, University of Edinburgh, and Nikolay Malkin, Chancellor's Fellow (AI and Datascience), School of Informatics, University of Edinburgh
This article is republished from The Conversation under a Creative Commons license. Read the original article.
What's Your Reaction?
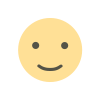
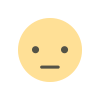
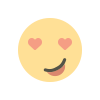

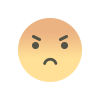
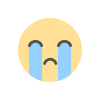
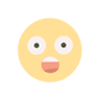