Driverless cars still lack common sense. AI chatbot technology could be the answer
New AI systems with language capabilities – such as the technology behind chatbots like ChatGPT – could be key to making driverless cars reason and behave more like human drivers

Estimated reading time: 9 minutes
A quick search on the internet will yield numerous videos showcasing the mishaps of driverless cars, often bringing a smile or laugh. But why do we find these behaviours amusing? It might be because they starkly contrast with how a human driver would handle similar situations.
Everyday situations that seem trivial to us can still pose significant challenges to driverless cars. This is because they are designed using engineering methods that differ fundamentally from how the human mind works. However, recent advancements in AI have opened up new possibilities.
Research on autonomous driving gained significant momentum in the late 2010s with the advent of deep neural networks (DNNs), a form of artificial intelligence (AI) that involves processing data in a way that is inspired by the human brain. This enables the processing of traffic scenario images and videos to identify “critical elements”, such as obstacles.
Detecting these often involves computing a 3D box to determine the sizes, orientations, and positions of the obstacles. This process, applied to vehicles, pedestrians and cyclists, for example, creates a representation of the world based on classes and spatial properties, including distance and speed relative to the driverless car.
This is the foundation of the most widely adopted engineering approach to autonomous driving, known as “sense-think-act”. In this approach, sensor data is first processed by the DNN. The sensor data is then used to predict obstacle trajectories. Finally the systems plan the car’s next actions.
While this approach offers benefits like easy debugging, the sense-think-act framework has a critical limitation: it is fundamentally different from the brain mechanisms behind human driving.
Lessons from the brain
Much about brain function remains unknown, making it challenging to apply intuition derived from the human brain to driverless vehicles. Nonetheless, various research efforts aim to take inspiration from neuroscience, cognitive science, and psychology to improve autonomous driving.
A long-established theory suggests that “sense” and “act” are not sequential but closely interrelated processes. Humans perceive their environment in terms of their capacity to act upon it.
For instance, when preparing to turn left at an intersection, a driver focuses on specific parts of the environment and obstacles relevant to the turn. In contrast, the sense-think-act approach processes the entire scenario independently of current action intentions.
Another critical difference with humans is that DNNs primarily rely on the data they have been trained on. When exposed to a slight unusual variation of a scenario, they might fail or miss important information.
Such rare, underrepresented scenarios, known as “long-tail cases”, present a major challenge. Current workarounds involve creating larger and larger training datasets, but the complexity and variability of real-life situations make it impossible to cover all possibilities.
As a result, data-driven approaches like sense-think-act struggle to generalise to unseen situations. Humans, on the other hand, excel at handling novel situations.
Thanks to a general knowledge of the world, we are able to assess new scenarios using “common sense”: a mix of practical knowledge, reasoning, and an intuitive understanding of how people generally behave, built from a lifetime of experiences.
In fact, driving for humans is another form of social interaction, and common sense is key to interpreting the behaviours of road users (other drivers, pedestrians, cyclists). This ability enables us to make sound judgments and decisions in unexpected situations.
Copying common sense
Replicating common sense in DNNs has been a significant challenge over the past decade, prompting scholars to call for a radical change in approach. Recent AI advancements are finally offering a solution.
Large language models (LLMs) are the technology behind chatbots such as ChatGPT and have demonstrated remarkable proficiency in understanding and generating human language. Their impressive abilities stem from being trained on vast amounts of information across various domains, which has allowed them to develop a form of common sense akin to ours.
More recently, multimodal LLMs (which can respond to user requests in text, vision and video) like GPT-4o and GPT-4o-mini have combined language with vision, integrating extensive world knowledge with the ability to reason about visual inputs.
These models can comprehend complex unseen scenarios, provide natural language explanations, and recommend appropriate actions, offering a promising solution to the long-tail problem.
In robotics, vision-language-action models (VLAMs) are emerging, combining linguistic and visual processing with actions from the robot. VLAMs are demonstrating impressive early results in controlling robotic arms through language instructions.
In autonomous driving, initial research is focusing on using multimodal models to provide driving commentary and explanations of motor planning decisions. For example, a model might indicate, “There is a cyclist in front of me, starting to decelerate,” providing insights into the decision-making process and enhancing transparency. The company Wayve has shown promising initial results in applying language-driven driverless cars at a commercial level.
Future of driving
While LLMs can address long-tail cases, they present new challenges. Evaluating their reliability and safety is more complex than for modular approaches like sense-think-act. Each component of an autonomous vehicle, including integrated LLMs, must be verified, requiring new testing methodologies tailored to these systems.
Additionally, multimodal LLMs are large and demanding on a computer’s resources, leading to high latency (a delay in action or communication from the computer). Driverless cars need real-time operation, and current models cannot generate responses quickly enough. Running LLMs also requires significant processing power and memory, which conflicts with the limited hardware constraints of vehicles.
Multiple research efforts are now focused on optimising LLMs for use in vehicles. It will take a few years before we see commercial driverless vehicles with common-sense reasoning on the streets.
However, the future of autonomous driving is bright. In AI models featuring language capabilities, we have a solid alternative to the sense-think-act paradigm, which is nearing its limits.
LLMs are widely considered the key to achieving vehicles that can reason and behave more like humans. This advancement is crucial, considering that approximately 1.19 million people die each year due to road traffic crashes.
Road traffic injuries are the leading cause of death for children and young adults aged 5-29 years. The development of autonomous vehicles with human-like reasoning could potentially reduce these numbers significantly, saving countless lives.
Alice Plebe, Research Fellow in Machine Intelligence, UCL
This article is republished from The Conversation under a Creative Commons license. Read the original article.
What's Your Reaction?
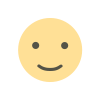
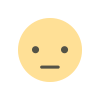
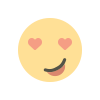

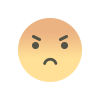
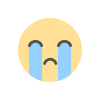
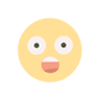