Machine learning is helping police work out what people on the run now look like
The Italian police recently arrested Matteo Messina Denaro, the alleged leader of the Sicilian Mafia who has been on the run since 1993. To aid in the search, the Carabinieri issued an artificially aged image to show what he might look like now

Estimated reading time: 8 minutes
Peter Hancock, University of Stirling and Charlie Frowd, University of Central Lancashire
Artists have traditionally made these images by altering old photos of the suspect – adding wrinkles, hair loss and other common aspects of ageing. But in recent years there has been a move towards using computer systems that employ machine learning, a much more sophisticated and formalised way of changing people’s faces.
We can’t predict with certainty how a person will look years after their last available photo, but these images can still help police. Here’s how the technology has developed.
An evolving skill
The first computerised method, which has now been superseded, used the difference between two average images. Photographs of a number of people (perhaps 30) who matched the target for age, sex and general colouration were combined to make an average “young” image.
Another older set produced the average “older” face. The computer calculated the difference between the two images – greying hair, wrinkles and other characteristics – and applied that difference to the young face image, producing one that looked older.
Whether or not it did actually look like the subject as an older person is another matter, but when one of us (Peter Hancock) tried it, it produced an image that did look very like his father, just without the NHS spectacles.
Renowned US psychologist Alice O'Toole from the University of Texas at Dallas, accidentally discovered another aspect of computerised ageing. She was attempting to produce automatic caricatures of people in 3D, by emphasising any differences between an individual face and an average of other people’s faces at the same age. She found that the caricatures looked older.
It seems that, as a rule, people get more distinctive with age – thin people become gaunt, and big noses become bigger. This highlights an important aspect of ageing – we all do it differently.
Two things affect how we age: our genetics and our environment. Working outdoors can cause the skin to age. Smoking and diet can also have strong effects. An artist attempting to produce an aged image may refer to pictures of relatives to see how age has affected them. Some computerised methods also attempt to do this.
The influence of nature and nurture can make it tricky to accurately predict how someone may look in the future. Our research with US psychologists Jim Lampinen at the University of Arkansas and Blake Erickson at Texas A&M University-San Antonio indicates that there are large differences in how artists prepare an age progression.
We found that averaging age progressions from different artists were as good as the single best image. Since it is unknown in advance which will be the best image, this seems like a good way to improve accuracy.
Making several different images of a person, with a few possible ways in which they could have aged, could be a promising alternative. For example, it could show them with different levels of hair loss.
The idea takes its cue from the way that facial composites of a suspect are created. These composites are computerised likenesses based on eyewitness accounts. This is done in the hope that someone will be able to name the face to the police, providing an investigation with a lead (that may or may not be correct).
Software packages such as E-Fit and EvoFit (which we developed) can generate different versions of the offender’s image with and without a beard, with headwear and other common disguises. They also have the ability to change age, weight, health and other age-related aspects of appearance.
New ways forward
Current computerised systems for ageing suspects based on old photos deploy deep neural networks, of the sort that are transforming the field of artificial intelligence. These are advanced computer systems that can be used for complex tasks and incorporate the ability to improve by learning from examples.
The networks are trained with, or shown, a large sample of pictures in pairs, showing the same person at two different ages, and then learn to do the mapping – producing an older image when they have been given the young one.
While such a system might just learn the average transformation of a face in terms of age, it is also capable of learning much more detail – for example, whether a certain sort of face will age in a particular way.
The results can be compared with known samples of faces and different researchers compete to minimise the differences between the predictions and the reality. The technology is easily deployed – there are even phone apps that will age your face, if you so wish.
Returning to the aged image of Matteo Messina Denaro, it’s intriguing to note that – in our analysis – a computer face recognition system (another type of deep neural networks) matched the arrest photo to the 30-year-old picture, but not to the artificially aged image.
Since a key role for computer face recognition – if society decides to accept it – could be to look for long-wanted people, it suggests that more research may be needed to establish the best way to do so.
Peter Hancock, Professor of Psychology, University of Stirling and Charlie Frowd, Professor of Forensic Psychology, University of Central Lancashire
This article is republished from The Conversation under a Creative Commons license. Read the original article.
What's Your Reaction?
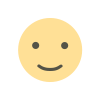
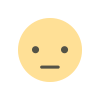
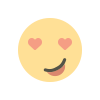

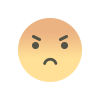
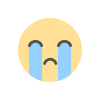
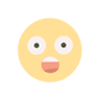